You are here: / Home
Optimizing HVAC operation with machine learning
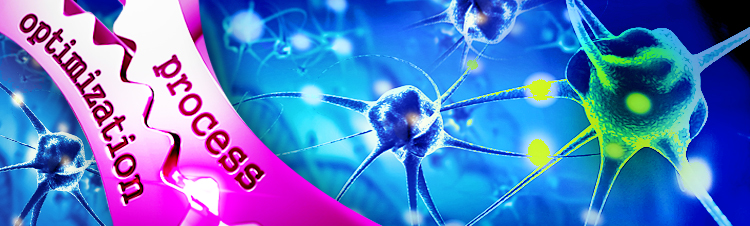
BMWi Euronorm Innokom
01/2019–05/2021
Dr.-Ing. Thomas Oppelt
+49-351-4081-5321
in progress
Intelligent control of HVAC systems – high comfort with low energy demand
Motivation
During operation, the energy efficiency of many HVAC systems remains considerably below the value predicted when planning. One reason is that especially complex systems with multiple generators, storages and consumer locations frequently are not operated optimally.
Aim of the project
Development of a tool for optimizing the operation of HVAC systems which uses machine learning (ML) methods and data from the digital building model (Building Information Model, BIM):
-
Optimization goal: high energy efficiency with at the same time high comfort for users
-
Saving operating costs, energy and carbon dioxide emissions due to increased efficiency
-
Continuous autonomous improvement of the ML algorithm by learning from new measured data with auto-adaptive reaction to changing conditions (building, system, use, smart meter for real time billing of energy and media, etc.)
Approach
- Reproduction of the real system’s thermal-energetic behaviour in the machine learning system, training with BIM data, measured data and a digital twin of the real system
-
Application of ML methods for load forecasting (weather, usage patterns)
-
Automatic classification of utilisation scenarios, fault detection
-
Integration of available tools for efficient simulation of indoor air flows and for calculating energy demands
-
Co-Validation of optimization tool, experimental studies and digital twin
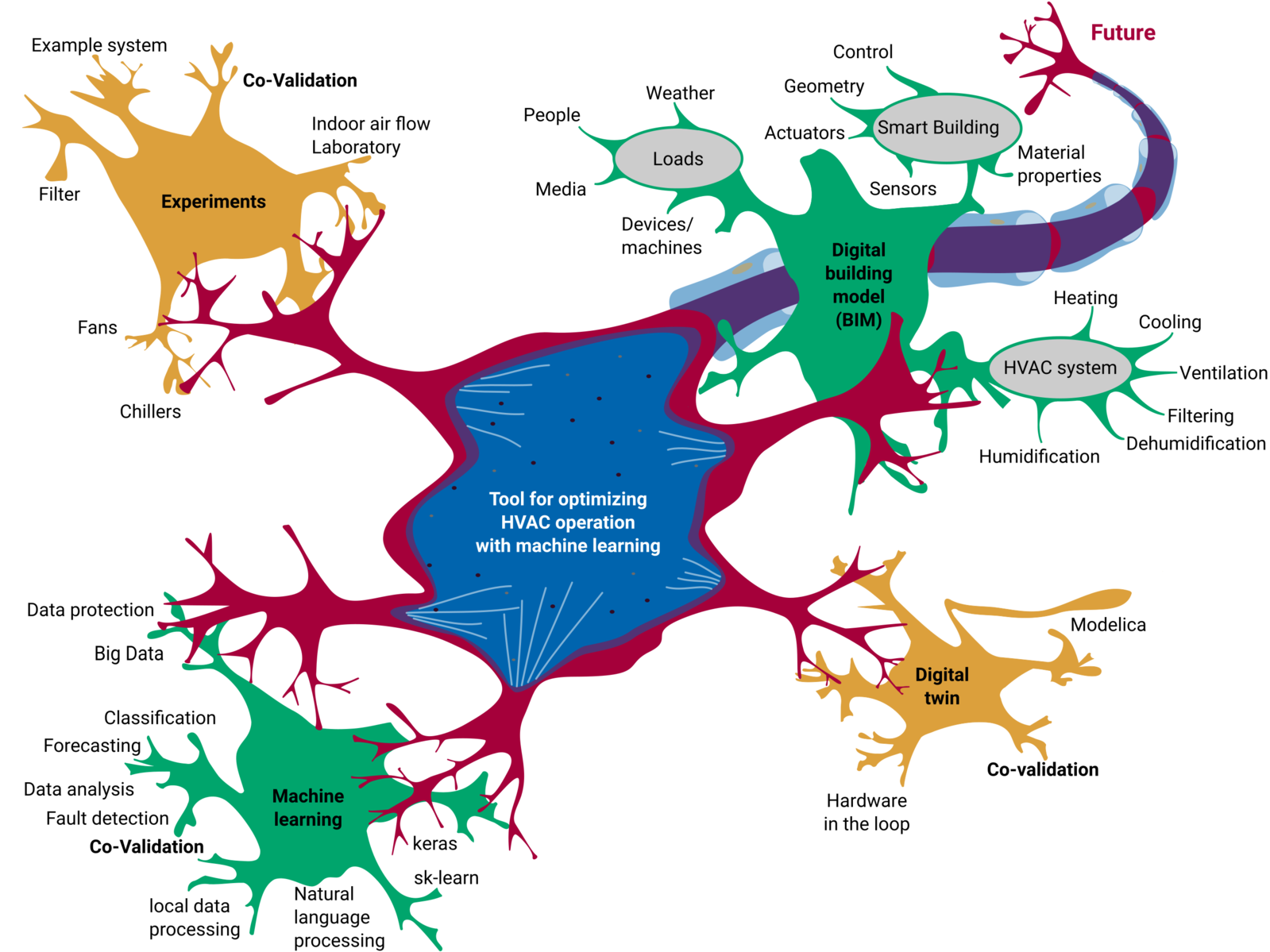
Interested?
Please get in touch with us if you are interested in a cooperation: klima@ilkdresden.de